By clicking “Accept All Cookies”, you agree to the storing of cookies on your device to enhance site navigation, analyze site usage, and assist in our marketing efforts Cookies Policy.
Architect of Engagement: Bhaskar Yakkanti and the Personalized Data Era
Beneath that convenience sits a rigorous ethics stack. Differential privacy guards low-cardinality segments; synthetic data augments sparse classes; and bias scans flank model re-training.
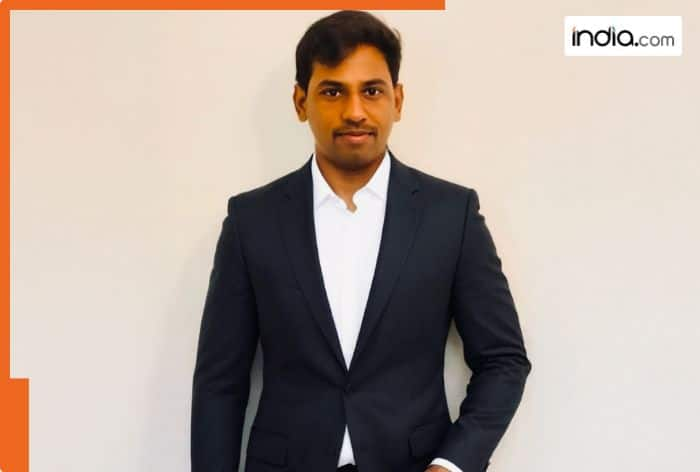
In the bustling universe of hospitality, guest expectations evolve minute by minute. Bhaskar Yakkanti’s Customer Data Platform (CDP)—deployed for a leading global hospitality-and-gaming group—answers that volatility with a lake-house architecture that fuses hotel bookings, gaming activity, event tickets, and on-property purchases into a single, privacy-hardened spine. Streaming collectors ingest five million events an hour, Spark enrichment jobs fuse behavioural traits with loyalty metadata, and the polished record lands in Azure Synapse views that marketers query in seconds. Revenue strategists can now retune campaign cohorts midway through a holiday weekend rather than waiting for next-day batches, a shift that clips acquisition spend by double-digit margins while lifting repeat-stay rates.
The platform’s backbone emerged from Bhaskar’s conviction that lineage must travel with every row. A Python validation framework performs schema drift checks before data reaches downstream marts; failed records detour to a quarantine route, preserving analytic integrity without slowing the happy path. Even compliance auditors—once tethered to protracted lineage hunts—trace a guest’s profile from kiosk swipe to marketing blast in fewer than ten clicks. The immediacy has reshaped decision cycles: revenue teams hold “lunch-and-launch” huddles that swap static weekly reports for real-time dashboards, and property managers experiment with room-upgrade incentives that react to occupancy swell in under thirty minutes.
“My experience of implementing large-scale data pipelines is that clarity in customer intent dictates every design choice, and once the ‘why’ is visible, the ‘how’ aligns naturally,” Bhaskar notes, underscoring that his architecture privileges business context over tooling hype.
Powering Instant Decisions in Financial Services
Long before casino floors and resort towers, Bhaskar refined his craft inside a global payments institution struggling with fraud-screening latency. Legacy MapR clusters digested card-swipe logs overnight, leaving investigators to chase stale anomalies. Bhaskar replaced the batch monolith with Spark Structured Streaming, partitioned on issuer geography and card family, then emitted micro-batches every three seconds to a rules-engine sandbox. Incident-response windows compressed from hours to minutes; false-positive escalations dropped by 22 percent; and the institution reclaimed seven figures in dispute fees during the first quarter post-cutover.
Equally transformative was his decision to lay observability rails before performance tuning. He instrumented Kafka topic lag, executor memory churn, and rule-evaluation latency in Grafana, letting SREs correlate user complaints with pipeline health in real time. That transparency shifted the culture from reactive war-room scrambles to proactive capacity planning: peak holiday shopping now triggers automated autoscaling rather than frantic hardware requisitions.
“Implementing enterprise streaming taught me that you can’t optimize what you can’t witness,” he tells colleagues. “Sustainable speed emerges only when telemetry, not adrenaline, guides the throttle.”
Engineering Cloud-Native Scalability Without Sacrificing Certainty
Bhaskar’s cloud playbook rejects blanket migration mantras in favour of selective control. In the CDP, transient Spark pools absorb promotional surges, yet deterministic SQL pools guard finance aggregates whose quarter-close deadlines brook no jitter. For legacy Teradata marts, he orchestrated phased lifts: first replicating tables to Delta Lake, then throttling dual-writes until cost-to-serve validated the switchover. The outcome: a 28 percent infrastructure-spend reduction and 40 percent faster analytics refresh, achieved without a single missed service-level objective.
Key to that success is Bhaskar’s “blue-green schema” principle. Every breaking change spawns a parallel dataset with full lineage tags, letting analysts A/B test queries while pipelines stabilize. Confluence runbooks codify cut-over timing, rollback triggers, and owner sign-offs—documents that slash onboarding time for new engineers and expand institutional memory.
“Years of leading cloud migrations convinced me that governance is not overhead; it is the receipt that lets leadership spend an insight with confidence,” he remarks, framing compliance as a value multiplier rather than a speed bump.
Building Governance and Trust into Every Byte
In Bhaskar’s view, data quality is inseparable from business credibility. His Python validation suite—deployed across finance, loyalty, and operations marts—executes column-level null-rate thresholds, referential integrity checks, and PII redaction before write-ahead logs commit. Alerting hooks into Microsoft Teams channel anomalies to domain owners, turning data stewardship from a back-office chore into a shared muscle.
He extends that ethos to security. Field-level encryption keys rotate via Azure Key Vault every 30 minutes; privilege boundaries mirror the company’s zero-trust macros; and access reviews auto-generate diff-reports for identity teams. When a hospitality subsidiary requested GDPR alignment, Bhaskar’s lineage mapping shaved the remediation estimate by half because sensitive fields were already traceable to their ingress points.
Finally, he threads ethical guardrails into machine-learning workflows. Model cards declare data provenance, training drift metrics, and fairness audits. Deployment pipelines block models lacking bias attestations, preventing “shadow AI” from reaching production. That diligence not only appeases regulators but also lends the brand a transparency halo coveted in the loyalty space.
Mentoring Engineers and Business Stakeholders Alike
Bhaskar multiplies impact by elevating those around him. A 12-week rotation cycles new hires through ingestion, transformation, and visualization squads; shadow commits mature into lead features by week nine. Attrition among graduates hovers below five percent, a stat HR attributes to his scaffolded learning path.
Yet mentorship extends beyond engineers. Product owners attend “data-design studios” where white-boarded user stories receive lineage annotations in real time. Finance analysts preview SQL plans before rollout, ensuring metric alignment. And quarterly “pipeline retros” invite marketing, risk, and compliance to grade data freshness, defect rates, and analytic adoption—an exercise that has lifted cross-team Net Promoter Scores by 18 points in two years.
The payoff surfaces during audits and performance reviews alike. Engineers present stack decisions in business vernacular, while executives reference lineage dashboards confidently in board decks. The cultural dividend: data ceases to be an IT asset and becomes a lingua franca that knits departments together.
AI-Driven Horizons: Closing the Loop from Creation to Consequence
Looking forward, Bhaskar envisions large-language-model copilots absorbing descriptive analytics so humans can hunt causal insight. He is already piloting a retrieval-augmented generation layer that condenses campaign metrics into prose directly inside the CRM, cutting weekly review prep from four hours to fifteen minutes.
Beneath that convenience sits a rigorous ethics stack. Differential privacy guards low-cardinality segments; synthetic data augments sparse classes; and bias scans flank model re-training. The coming wave, Bhaskar predicts, is vector databases that store behavioural embeddings alongside transactional facts, powering room-upgrade nudges the moment a guest slows at a lobby kiosk. But precision must never outpace permission: consent flags propagate through Kafka headers, ensuring downstream models honour opt-outs instantly.
“Scaling machine learning has shown me that relevance without trust is thin ice; only when transparency walks in lockstep with automation does AI create durable value,” Bhaskar contends, summarizing a philosophy that weds innovation to accountability.
For breaking news and live news updates, like us on Facebook or follow us on Twitter and Instagram. Read more on Latest Money News on India.com.